Inference with clouds aware floods segmentation model#
Last Modified: 30-11-2023
Authors: Gonzalo Mateo-García, Enrique Portalés-Julià
This notebook shows how to load and make inferences with the clouds aware flood segmentation model proposed in:
E. Portalés-Julià, G. Mateo-García, C. Purcell, and L. Gómez-Chova Global flood extent segmentation in optical satellite images. Scientific Reports 13, 20316 (2023). DOI: 10.1038/s41598-023-47595-7.
With this model we are able to correctly classify land/water in partially cloud covered places and over thin and semi-transparent clouds.
ml4floods trained model#
The following scheme details the training and inference workflow of the WorldFloods multioutput models. We will load the trained model into a function that outputs a 3-class prediction by applying prediction rule detailed below:
import os
from huggingface_hub import hf_hub_download, hf_hub_url
import torch
import numpy as np
from shapely.geometry import shape
from georeader.rasterio_reader import RasterioReader
from georeader.geotensor import GeoTensor
from georeader.readers import ee_query
from georeader.readers import ee_image
from datetime import datetime
from georeader import plot
import ee
from ml4floods.visualization import plot_utils
import matplotlib.pyplot as plt
COLORS_PRED = np.array([[0, 0, 0], # 0: invalid
[139, 64, 0], # 1: land
[0, 0, 240], # 2: water
[220, 220, 220], # 3: cloud
[60, 85, 92]], # 5: flood_trace
dtype=np.float32) / 255
Load trained model#
Read the trained model from our HuggingFace repo. We will load the Unet multioutput S2-to-L8 model of the paper. In case you want to load a different model set the experiment_name
variable below to according to:
Unet multioutput -
WF2_unetv2_all
Unet multioutput S2-to-L8 -
WF2_unetv2_bgriswirs
Unet multioutput RGBNIR -
WF2_unetv2_rgbi
experiment_name = "WF2_unetv2_bgriswirs"
subfolder_local = f"models/{experiment_name}"
config_file = hf_hub_download(repo_id="isp-uv-es/ml4floods",subfolder=subfolder_local, filename="config.json",
local_dir=".", local_dir_use_symlinks=False)
model_file = hf_hub_download(repo_id="isp-uv-es/ml4floods",subfolder=subfolder_local, filename="model.pt",
local_dir=".", local_dir_use_symlinks=False)
from ml4floods.scripts.inference import load_inference_function
from ml4floods.models.model_setup import get_channel_configuration_bands
inference_function, config = load_inference_function(subfolder_local, device_name = 'cpu', max_tile_size=1024,
th_water=0.7, th_brightness=3500,
distinguish_flood_traces=True)
channel_configuration = config['data_params']['channel_configuration']
channels = get_channel_configuration_bands(channel_configuration, collection_name='S2')
Loaded model weights: models/WF2_unetv2_bgriswirs/model.pt
Getting model inference function
def predict(input_tensor, channels = [1, 2, 3, 7, 11, 12] ):
input_tensor = input_tensor.astype(np.float32)
input_tensor = input_tensor[channels]
torch_inputs = torch.tensor(np.nan_to_num(input_tensor))
return inference_function(torch_inputs)
Inference on S2 data from the WorldFloodsv2 dataset#
The WorldFloodsv2 dataset is stored in this HuggingFace repository. We’ll grab a S2 image from the dataset and run inference on them.
# Select image to use
subset = "test"
filename = "EMSR264_18MIANDRIVAZODETAIL_DEL_v2"
s2url = hf_hub_url(repo_id="isp-uv-es/WorldFloodsv2",
subfolder=f"{subset}/S2", filename=f"{filename}.tif",
repo_type="dataset")
channels = get_channel_configuration_bands(channel_configuration, collection_name='S2')
s2rst = RasterioReader(s2url).isel({"band": channels})
s2rst = s2rst.load()
prediction_test, prediction_test_cont = predict(s2rst.values, channels = list(range(len(channels))))
prediction_test_raster = GeoTensor(prediction_test.numpy(), transform=s2rst.transform,
fill_value_default=0, crs=s2rst.crs)
prediction_test_raster
Transform: | 10.00, 0.00, 537430.00|
| 0.00,-10.00, 7844180.00|
| 0.00, 0.00, 1.00|
Shape: (1313, 1530)
Resolution: (10.0, 10.0)
Bounds: (537430.0, 7831050.0, 552730.0, 7844180.0)
CRS: EPSG:32738
fill_value_default: 0
fig, ax = plt.subplots(1,2,figsize=(14,7), sharey=True)
plot.show((s2rst.isel({"band": [4,3,2]})/3_500).clip(0,1), ax=ax[0], add_scalebar=True)
ax[0].set_title(f"{subset}/{filename}")
plot.plot_segmentation_mask(prediction_test_raster, COLORS_PRED, ax=ax[1],
interpretation_array=["invalids", "land", "water", "cloud", "flood_trace"])
ax[1].set_title(f"{subset}/{filename} floodmap")
Text(0.5, 1.0, 'test/EMSR264_18MIANDRIVAZODETAIL_DEL_v2 floodmap')
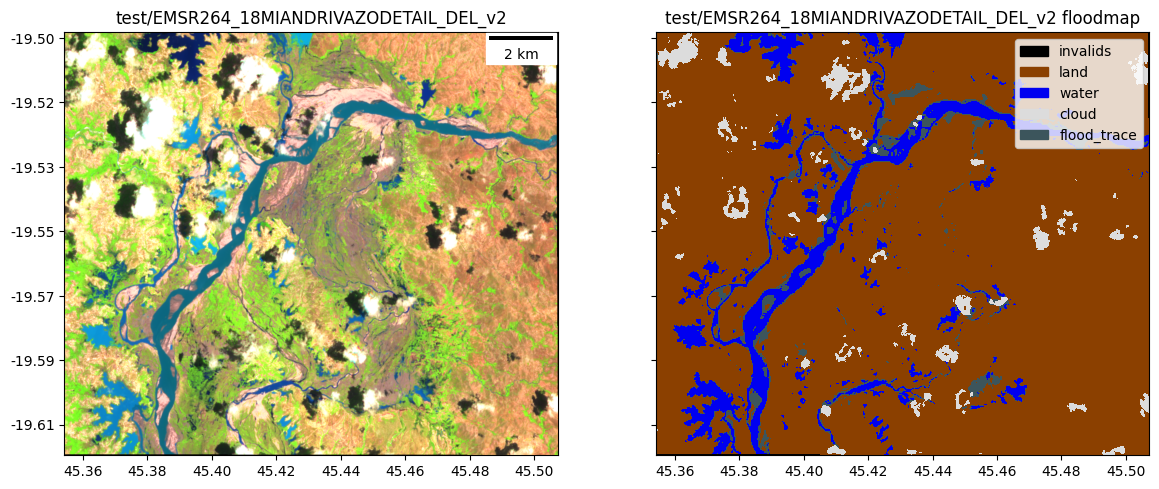
Inference on a new Sentinel-2 image#
Download a Sentinel-2 image#
ee.Authenticate()
To authorize access needed by Earth Engine, open the following URL in a web browser and follow the instructions:
The authorization workflow will generate a code, which you should paste in the box below.
Successfully saved authorization token.
# ee.Authenticate()
ee.Initialize()
aoi = shape({'type': 'Polygon',
'coordinates': [[[153.20789834941638, -28.75874177524779],
[153.20789834941638, -28.91332819718112],
[153.38848611797107, -28.91332819718112],
[153.38848611797107, -28.75874177524779]]]})
s2data = ee_query.query(aoi, datetime(2022,3,1), datetime(2022,3,2), producttype="S2")
bands_s2 = get_channel_configuration_bands(channel_configuration, collection_name='S2',as_string=True)
asset_id = f"{s2data.iloc[0].collection_name}/{s2data.iloc[0].gee_id}"
geom = s2data.iloc[0].geometry.intersection(aoi)
postflood = ee_image.export_image_getpixels(asset_id, geom, proj=s2data.iloc[0].proj,bands_gee=bands_s2)
postflood
/home/gonzalo/mambaforge/envs/ml4floods2/lib/python3.10/site-packages/geopandas/geoseries.py:645: FutureWarning: the convert_dtype parameter is deprecated and will be removed in a future version. Do ``ser.astype(object).apply()`` instead if you want ``convert_dtype=False``.
result = super().apply(func, convert_dtype=convert_dtype, args=args, **kwargs)
Warning 1: TIFFReadDirectory:Sum of Photometric type-related color channels and ExtraSamples doesn't match SamplesPerPixel. Defining non-color channels as ExtraSamples.
Warning 1: TIFFReadDirectory:Sum of Photometric type-related color channels and ExtraSamples doesn't match SamplesPerPixel. Defining non-color channels as ExtraSamples.
Warning 1: TIFFReadDirectory:Sum of Photometric type-related color channels and ExtraSamples doesn't match SamplesPerPixel. Defining non-color channels as ExtraSamples.
Warning 1: TIFFReadDirectory:Sum of Photometric type-related color channels and ExtraSamples doesn't match SamplesPerPixel. Defining non-color channels as ExtraSamples.
Transform: | 10.00, 0.00, 520260.00|
| 0.00,-10.00, 6818730.00|
| 0.00, 0.00, 1.00|
Shape: (6, 1717, 1766)
Resolution: (10.0, 10.0)
Bounds: (520260.0, 6801560.0, 537920.0, 6818730.0)
CRS: EPSG:32756
fill_value_default: 0.0
Run inference#
%%time
prediction_postflood, prediction_postflood_cont = predict(postflood.values, channels = list(range(len(bands_s2))))
prediction_postflood_raster = GeoTensor(prediction_postflood.numpy(), transform=postflood.transform,
fill_value_default=0, crs=postflood.crs)
CPU times: user 1min 30s, sys: 15.9 s, total: 1min 46s
Wall time: 14 s
Plot results#
fig, ax = plt.subplots(1,2,figsize=(14,7))
plot.show((postflood.isel({"band": [4,3,2]})/3_500).clip(0,1), ax=ax[0], add_scalebar=True)
ax[0].set_title(f"{s2data.iloc[0].satellite} {s2data.iloc[0].solarday}")
plot.plot_segmentation_mask(prediction_postflood_raster, COLORS_PRED, ax=ax[1],
interpretation_array=["invalids", "land", "water", "cloud", "flood_trace"])
ax[1].set_title(f"{s2data.iloc[0].solarday} floodmap")
Text(0.5, 1.0, '2022-03-02 floodmap')
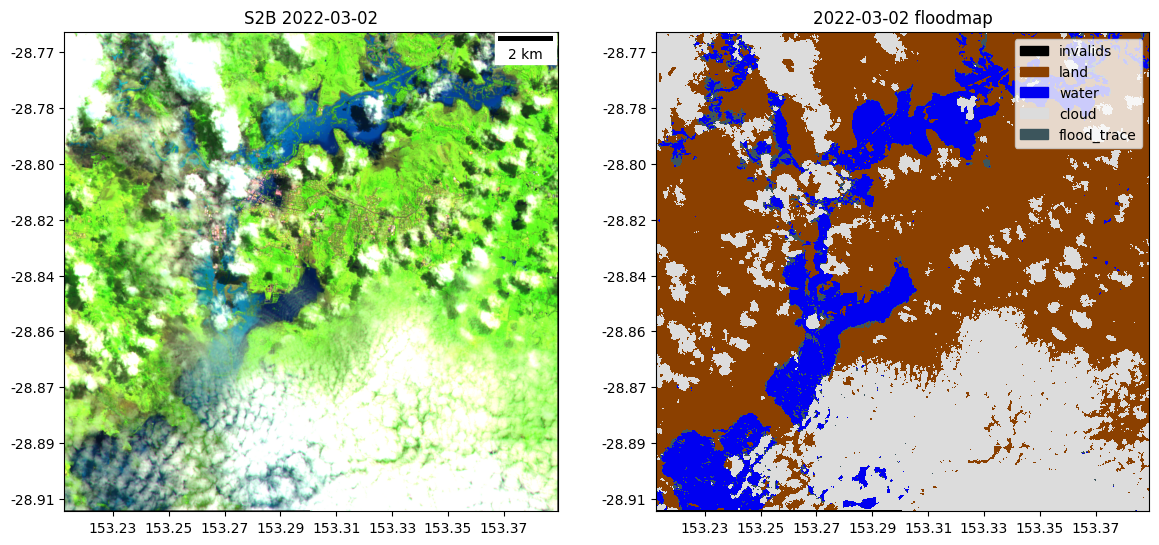
Inference on a new Landsat image#
Download a Landsat image#
%%time
satdata = ee_query.query(aoi, datetime(2022,4,4), datetime(2022,4,5), producttype="Landsat")
bands_landsat = get_channel_configuration_bands(channel_configuration, collection_name='Landsat',as_string=True)
asset_id = f"{satdata.iloc[0].collection_name}/{satdata.iloc[0].gee_id}"
geom = satdata.iloc[0].geometry.intersection(aoi)
postfloodl8 = ee_image.export_image_getpixels(asset_id, geom, proj=satdata.iloc[0].proj,bands_gee=bands_landsat)
postfloodl8.values *= 10000
postfloodl8
/home/gonzalo/mambaforge/envs/ml4floods2/lib/python3.10/site-packages/geopandas/geoseries.py:645: FutureWarning: the convert_dtype parameter is deprecated and will be removed in a future version. Do ``ser.astype(object).apply()`` instead if you want ``convert_dtype=False``.
result = super().apply(func, convert_dtype=convert_dtype, args=args, **kwargs)
CPU times: user 148 ms, sys: 15.2 ms, total: 163 ms
Wall time: 4.47 s
Warning 1: TIFFReadDirectory:Sum of Photometric type-related color channels and ExtraSamples doesn't match SamplesPerPixel. Defining non-color channels as ExtraSamples.
Transform: | 30.00, 0.00, 520245.00|
| 0.00,-30.00,-3181245.00|
| 0.00, 0.00, 1.00|
Shape: (6, 573, 590)
Resolution: (30.0, 30.0)
Bounds: (520245.0, -3198435.0, 537945.0, -3181245.0)
CRS: EPSG:32656
fill_value_default: -inf
Run inference#
%%time
prediction_postfloodl8, prediction_postflood_contl8 = predict(postfloodl8.values, channels = list(range(len(bands_s2))))
prediction_postfloodl8_raster = GeoTensor(prediction_postfloodl8.numpy(), transform=postfloodl8.transform,
fill_value_default=0, crs=postfloodl8.crs)
prediction_postfloodl8_raster
CPU times: user 9.22 s, sys: 1.38 s, total: 10.6 s
Wall time: 1.38 s
Transform: | 30.00, 0.00, 520245.00|
| 0.00,-30.00,-3181245.00|
| 0.00, 0.00, 1.00|
Shape: (573, 590)
Resolution: (30.0, 30.0)
Bounds: (520245.0, -3198435.0, 537945.0, -3181245.0)
CRS: EPSG:32656
fill_value_default: 0
Plot results#
fig, ax = plt.subplots(1,2,figsize=(14,7))
plot.show((postfloodl8.isel({"band": [4,3,2]})/3_500).clip(0,1), ax=ax[0], add_scalebar=True)
ax[0].set_title(f"{satdata.iloc[0].satellite} {satdata.iloc[0].solarday}")
plot.plot_segmentation_mask(prediction_postfloodl8_raster, COLORS_PRED, ax=ax[1],
interpretation_array=["invalids", "land", "water", "cloud", "flood_trace"])
ax[1].set_title(f"{satdata.iloc[0].solarday} floodmap")
Text(0.5, 1.0, '2022-04-05 floodmap')
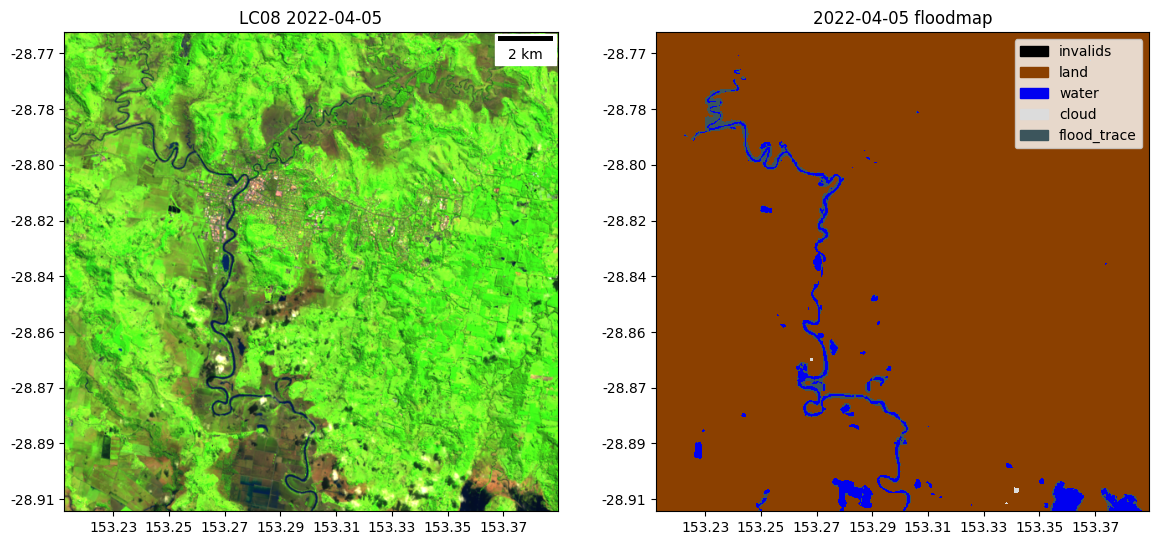
More examples reading data from the GCP bucket#
This requires setting up a project to read from our publicly available gcp bucket
os.environ["GOOGLE_APPLICATION_CREDENTIALS"] = "/path/to/gcc_pays.json"
os.environ['GS_USER_PROJECT']= 'your-project'
os.environ['CUDA_VISIBLE_DEVICES'] = '0'
Step 2: Helper functions for plotting and reading some demo data#
Show code cell source
import rasterio
import numpy as np
from rasterio import plot as rasterioplt
import matplotlib.pyplot as plt
from matplotlib import colors
import matplotlib.patches as mpatches
from typing import Optional, Tuple, Union
import torch
from ml4floods.data.worldfloods.configs import BANDS_S2
from ml4floods.visualization.plot_utils import plot_segmentation_mask
@torch.no_grad()
def read_inference_pair(tiff_inputs:str, folder_ground_truth:str,
window:Optional[Union[rasterio.windows.Window, Tuple[slice,slice]]],
return_ground_truth: bool=False, channels:bool=None,
folder_permanent_water=Optional[str],
cache_folder=None) -> Tuple[torch.Tensor, torch.Tensor, torch.Tensor, rasterio.Affine]:
"""
Read a pair of layers from the worldfloods bucket and return them as Tensors to pass to a model, return the transform for plotting with lat/long
Args:
tiff_inputs: filename for layer in worldfloods bucket
folder_ground_truth: folder name to be replaced by S2 in the input
window: window of layer to use
return_ground_truth: flag to indicate if paired gt layer should be returned
channels: list of channels to read from the image
return_permanent_water: Read permanent water layer raster
Returns:
(torch_inputs, torch_targets, transform): inputs Tensor, gt Tensor, transform for plotting with lat/long
"""
if cache_folder is not None and tiff_inputs.startswith("gs"):
tiff_inputs = download_tiff(cache_folder, tiff_inputs, folder_ground_truth, folder_permanent_water)
tiff_targets = tiff_inputs.replace("/S2/", folder_ground_truth)
with rasterio.open(tiff_inputs, "r") as rst:
inputs = rst.read((np.array(channels) + 1).tolist(), window=window)
# Shifted transform based on the given window (used for plotting)
transform = rst.transform if window is None else rasterio.windows.transform(window, rst.transform)
torch_inputs = torch.Tensor(inputs.astype(np.float32)).unsqueeze(0)
if folder_permanent_water is not None:
tiff_permanent_water = tiff_inputs.replace("/S2/", folder_permanent_water)
with rasterio.open(tiff_permanent_water, "r") as rst:
permanent_water = rst.read(1, window=window)
torch_permanent_water = torch.tensor(permanent_water)
else:
torch_permanent_water = torch.zeros_like(torch_inputs)
if return_ground_truth:
with rasterio.open(tiff_targets, "r") as rst:
targets = rst.read(1, window=window)
torch_targets = torch.tensor(targets).unsqueeze(0)
else:
torch_targets = torch.zeros_like(torch_inputs)
return torch_inputs, torch_targets, torch_permanent_water, transform
COLORS_WORLDFLOODS = np.array([[0, 0, 0], # invalid
[139, 64, 0], # land
[0, 0, 139], # water
[220, 220, 220]], # cloud
dtype=np.float32) / 255
INTERPRETATION_WORLDFLOODS = ["invalid", "land", "water", "cloud"]
COLORS_WORLDFLOODS_PERMANENT = np.array([[0, 0, 0], # 0: invalid
[139, 64, 0], # 1: land
[237, 0, 0], # 2: flood_water
[220, 220, 220], # 3: cloud
[0, 0, 139], # 4: permanent_water
[60, 85, 92]], # 5: seasonal_water
dtype=np.float32) / 255
INTERPRETATION_WORLDFLOODS_PERMANENT = ["invalid", "land", "flood water", "cloud", "permanent water", "seasonal water"]
def gt_with_permanent_water(gt: np.ndarray, permanent_water: np.ndarray)->np.ndarray:
""" Permanent water taken from: https://developers.google.com/earth-engine/datasets/catalog/JRC_GSW1_2_YearlyHistory"""
gt[(gt == 2) & (permanent_water == 3)] = 4 # set as permanent_water
gt[(gt == 2) & (permanent_water == 2)] = 5 # set as seasonal water
return gt
def get_cmap_norm_colors(color_array, interpretation_array):
cmap_categorical = colors.ListedColormap(color_array)
norm_categorical = colors.Normalize(vmin=-.5,
vmax=color_array.shape[0]-.5)
patches = []
for c, interp in zip(color_array, interpretation_array):
patches.append(mpatches.Patch(color=c, label=interp))
return cmap_categorical, norm_categorical, patches
def plot_inference_set(inputs: torch.Tensor, targets: torch.Tensor,
predictions: torch.Tensor, permanent_water: torch.Tensor, transform: rasterio.Affine)->None:
"""
Plots inputs, targets and prediction into lat/long visualisation
Args:
inputs: input Tensor
targets: gt target Tensor
prediction: predictions output by model (softmax, argmax already applied)
permanent_water: permanent water raster
transform: transform used to plot with lat/long
"""
fig, ax = plt.subplots(2,2,figsize=(16,16))
inputs_show = inputs.cpu().numpy().squeeze()
targets_show = targets.cpu().numpy().squeeze()
permanent_water_show = permanent_water.numpy().squeeze()
targets_show = gt_with_permanent_water(targets_show, permanent_water_show)
# Color categories {-1: invalid, 0: land, 1: water, 2: clouds}
cmap_preds, norm_preds, patches_preds = get_cmap_norm_colors(COLORS_WORLDFLOODS, INTERPRETATION_WORLDFLOODS)
cmap_gt, norm_gt, patches_gt = get_cmap_norm_colors(COLORS_WORLDFLOODS_PERMANENT, INTERPRETATION_WORLDFLOODS_PERMANENT)
prediction_show = (predictions).cpu().numpy()
band_names_current_image = [BANDS_S2[iband] for iband in channels]
bands_rgb = [band_names_current_image.index(b) for b in ["B4", "B3", "B2"]] # swir_1, nir, red composite
bands_false_composite = [band_names_current_image.index(b) for b in ["B11", "B8", "B4"]] # swir_1, nir, red composite
false_rgb = np.clip(inputs_show[bands_false_composite, :, :]/3000.,0,1)
rgb = np.clip(inputs_show[bands_rgb, :, :]/3000.,0,1)
rasterioplt.show(rgb,transform=transform,ax=ax[0,0])
ax[0,0].set_title("RGB Composite")
rasterioplt.show(false_rgb,transform=transform,ax=ax[0,1])
ax[0,1].set_title("SWIR1,NIR,R Composite")
plot_segmentation_mask(targets_show,transform=transform, ax = ax[1,0],
color_array=COLORS_WORLDFLOODS_PERMANENT, interpretation_array=INTERPRETATION_WORLDFLOODS_PERMANENT)
plot_segmentation_mask(prediction_show,transform=transform, ax = ax[1,1], color_array=COLORS_WORLDFLOODS, interpretation_array=INTERPRETATION_WORLDFLOODS)
ax[1,0].set_title("Ground Truth")
ax[1,0].legend(handles=patches_gt,
loc='upper right')
ax[1,1].set_title("Prediction water")
ax[1,1].legend(handles=patches_preds,
loc='upper right')
Perform Inference using the inference_function
#
from ml4floods.models.model_setup import get_channel_configuration_bands
download_image = False
cache_folder = None # "tiffs_for_inference"
# os.makedirs(cache_folder, exist_ok=True)
tiff_s2, window, channels = "gs://ml4floods/worldfloods/public/test/S2/EMSR333_02PORTOPALO_DEL_MONIT01_v1_observed_event_a.tif", (slice(1000,None),slice(0,400)), get_channel_configuration_bands(config.model_params.hyperparameters.channel_configuration)
# Load the image and ground truth
torch_inputs, torch_targets, torch_permanent_water, transform = read_inference_pair(tiff_s2,folder_ground_truth="/gt/",
window=window, return_ground_truth=True, channels=channels,
folder_permanent_water="/PERMANENTWATERJRC/",
cache_folder=cache_folder)
# Compute the prediction
outputs, cont_pred = inference_function(torch_inputs[0]) # 3 class prediction with (h, w)
plot_inference_set(torch_inputs, torch_targets, outputs, torch_permanent_water, transform)
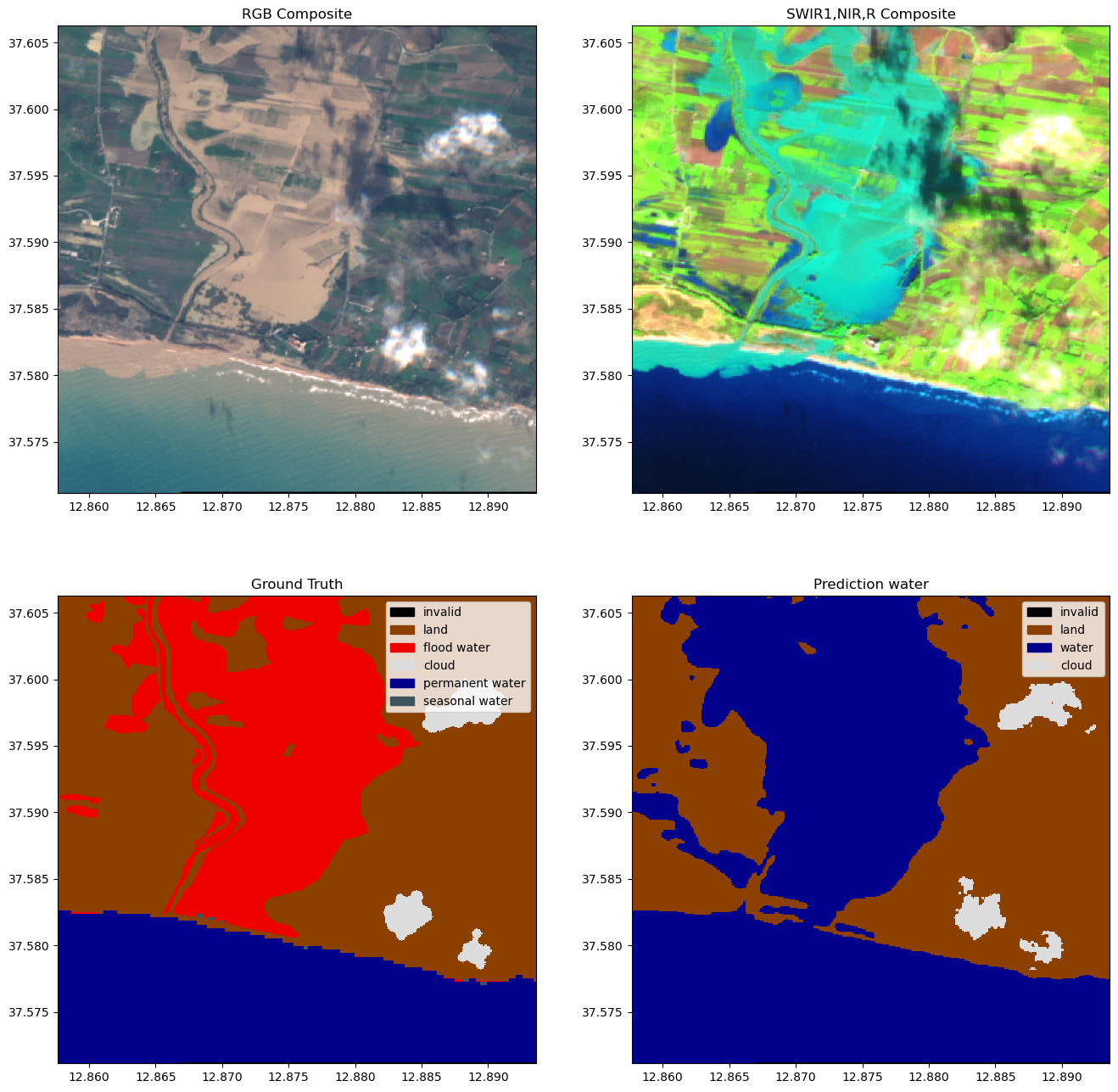
Lets try another image!#
import rasterio.windows
window = rasterio.windows.Window(col_off=4_860, row_off=3_300,
width=840, height=1000)
tiff_s2, channels = "gs://ml4cc_data_lake/2_PROD/2_Mart/worldfloods_v1_0/test/S2/EMSR342_06NORTHNORMANTON_DEL_v1_observed_event_a.tif", get_channel_configuration_bands(config.model_params.hyperparameters.channel_configuration)
torch_inputs, torch_targets, torch_permanent_water, transform = read_inference_pair(tiff_s2, folder_ground_truth="/gt/",
window=window,
return_ground_truth=True, channels=channels,
folder_permanent_water="/PERMANENTWATERJRC/",
cache_folder=None)
outputs, cont_pred = inference_function(torch_inputs[0]) # 3 class prediction with (h, w)
plot_inference_set(torch_inputs, torch_targets, outputs, torch_permanent_water, transform)
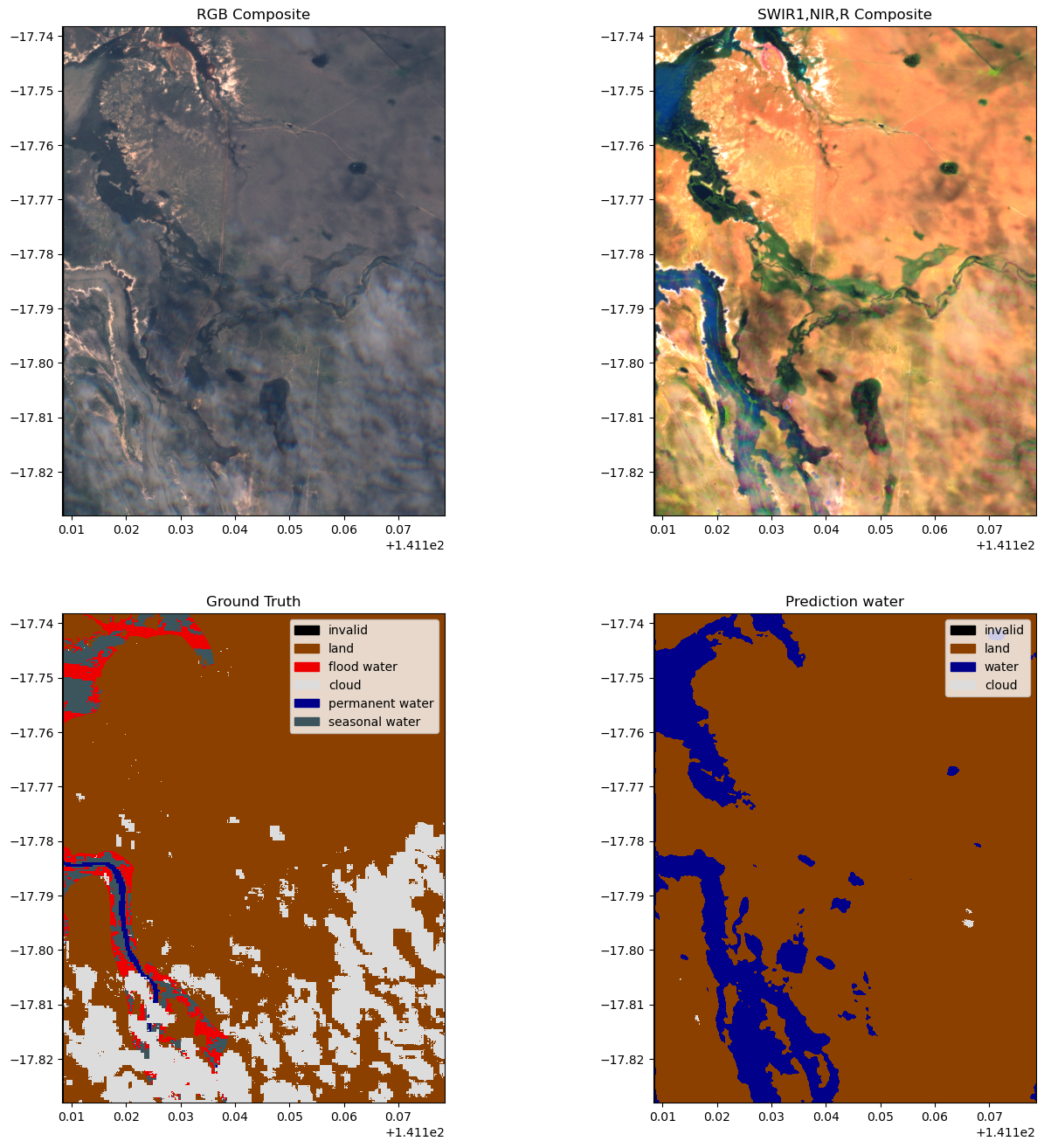
Lets try another image!#
import rasterio.windows
window = rasterio.windows.Window(col_off=1_600, row_off=400,
width=1000, height=1000)
tiff_s2, channels = "gs://ml4cc_data_lake/2_PROD/2_Mart/worldfloods_v1_0/val/S2/EMSR271_02FARKADONA_DEL_v1_observed_event_a.tif", get_channel_configuration_bands(config.model_params.hyperparameters.channel_configuration)
torch_inputs, torch_targets, torch_permanent_water, transform = read_inference_pair(tiff_s2, folder_ground_truth="/gt/",
window=window,
return_ground_truth=True, channels=channels,
folder_permanent_water="/PERMANENTWATERJRC/",
cache_folder=None)
outputs, cont_pred = inference_function(torch_inputs[0]) # 3 class prediction with (h, w)
plot_inference_set(torch_inputs, torch_targets, outputs, torch_permanent_water, transform)
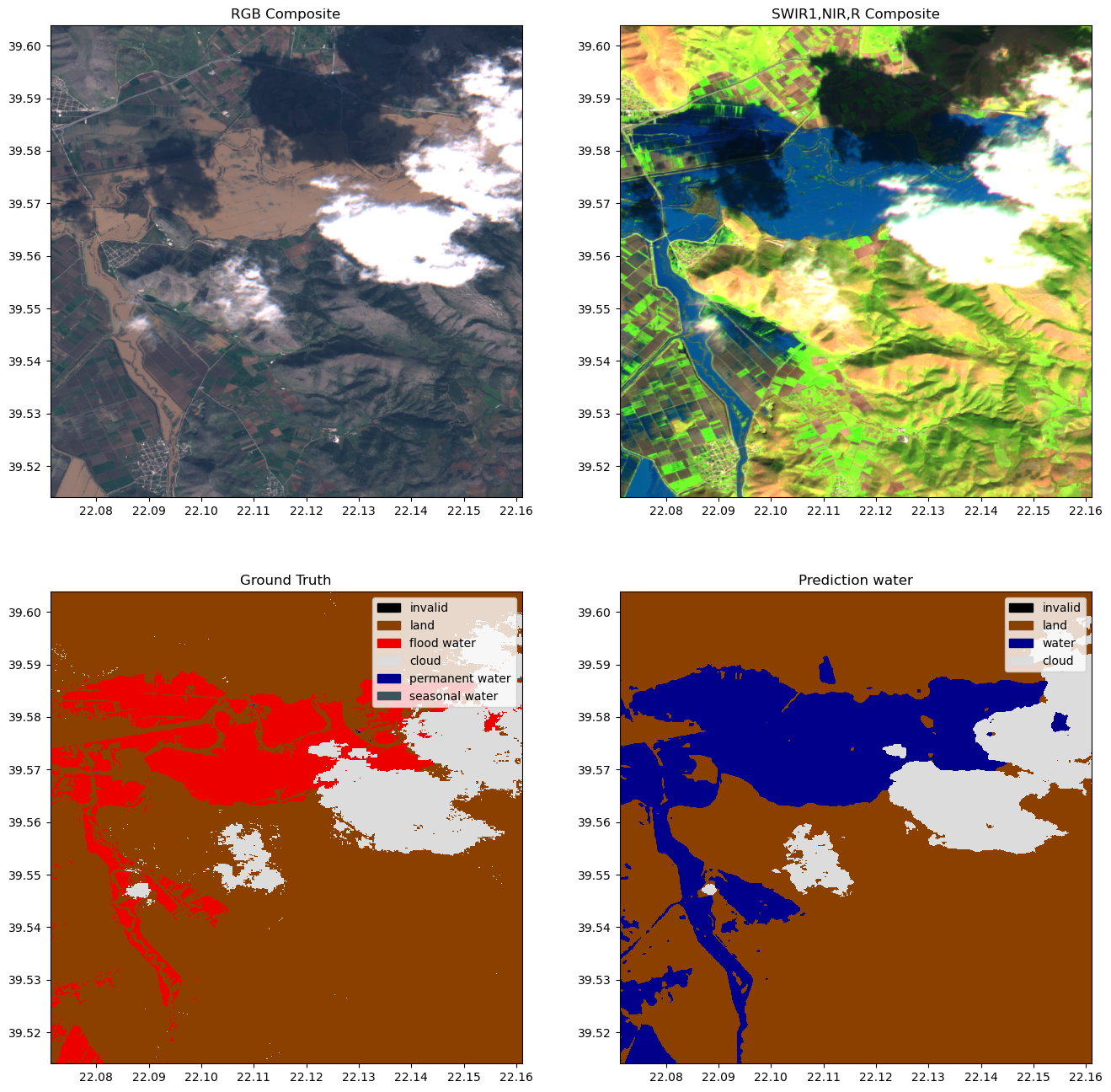
Lets try another image!#
window = None
tiff_s2, channels = "gs://ml4cc_data_lake/2_PROD/2_Mart/worldfloods_v1_0/S2/RS2_20161008_Water_Extent_Corail_Pestel.tif", get_channel_configuration_bands(config.model_params.hyperparameters.channel_configuration)
torch_inputs, torch_targets, torch_permanent_water, transform = read_inference_pair(tiff_s2, folder_ground_truth="/gt/",
window=None,
return_ground_truth=True, channels=channels,
folder_permanent_water="/PERMANENTWATERJRC/",
cache_folder=None)
outputs, cont_pred = inference_function(torch_inputs[0]) # 3 class prediction with (h, w)
plot_inference_set(torch_inputs, torch_targets, outputs, torch_permanent_water, transform)
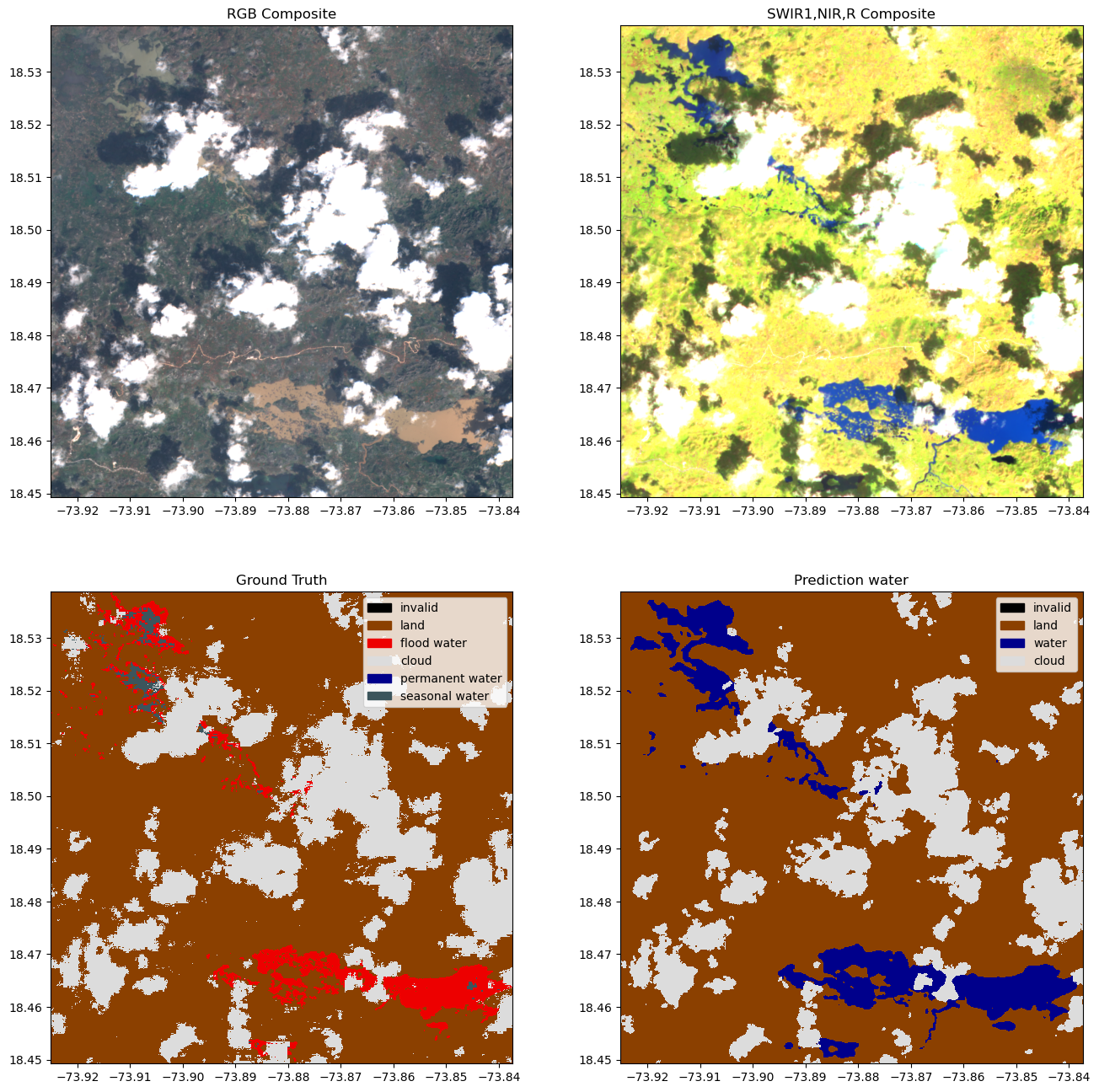
Lets try another image!#
import rasterio.windows
window = rasterio.windows.Window(col_off=0, row_off=1_200,
width=1000, height=1_500)
tiff_s2, channels = "gs://ml4cc_data_lake/2_PROD/2_Mart/worldfloods_v1_0/val/S2/ST1_20161014_WaterExtent_BinhDinh_Lake.tif", get_channel_configuration_bands(config.model_params.hyperparameters.channel_configuration)
torch_inputs, torch_targets, torch_permanent_water, transform = read_inference_pair(tiff_s2, folder_ground_truth="/gt/",
window=window,
return_ground_truth=True, channels=channels,
folder_permanent_water="/PERMANENTWATERJRC/",
cache_folder=None)
outputs, cont_pred = inference_function(torch_inputs[0])
plot_inference_set(torch_inputs, torch_targets, outputs, torch_permanent_water, transform)
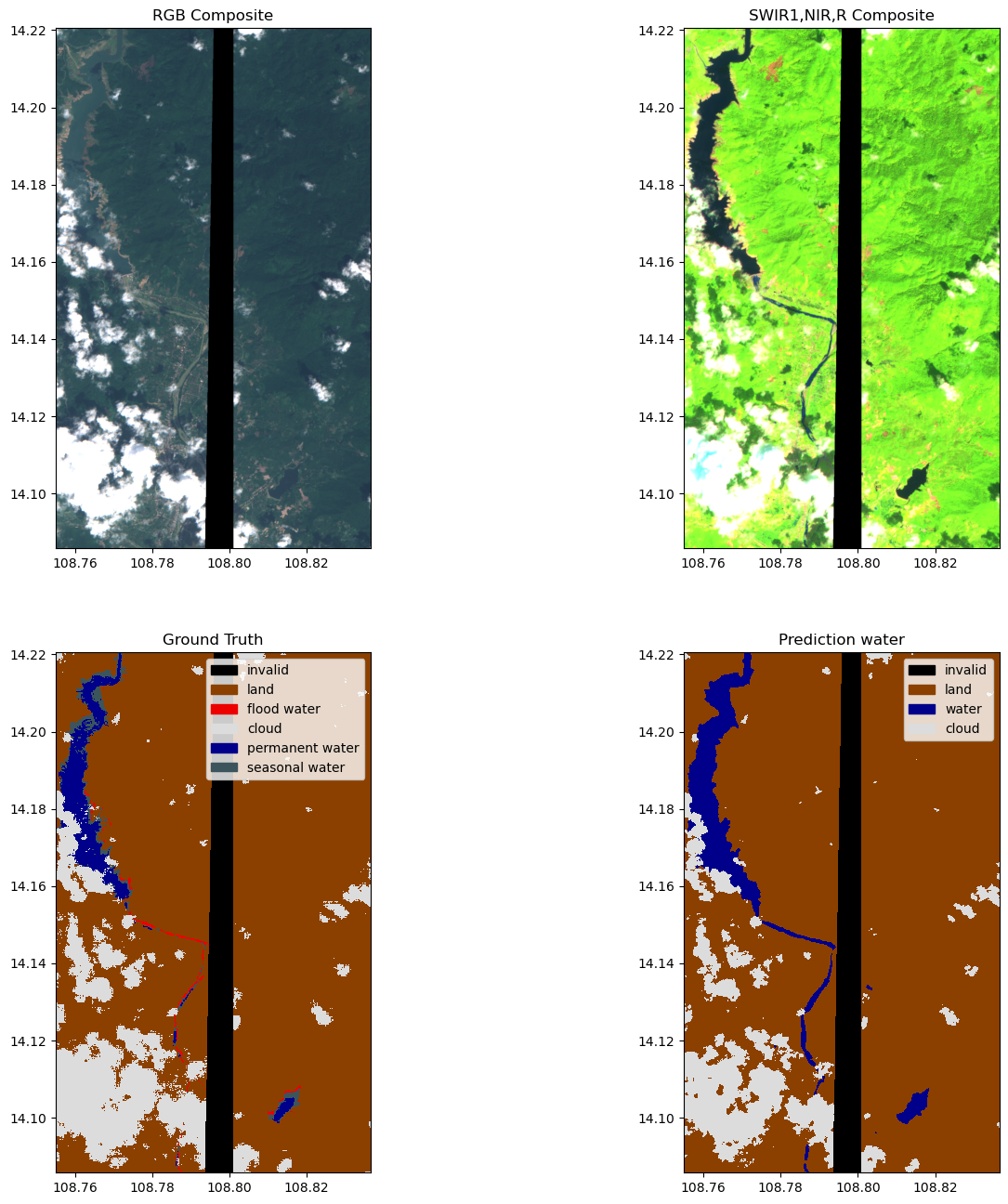
Lets try another image!#
import rasterio.windows
window = rasterio.windows.Window(col_off=0, row_off=0,
width=1_500, height=1_500)
tiff_s2, channels = "gs://ml4cc_data_lake/2_PROD/2_Mart/worldfloods_v1_0/test/S2/EMSR347_07ZOMBA_DEL_MONIT01_v1_observed_event_a.tif", get_channel_configuration_bands(config.model_params.hyperparameters.channel_configuration)
torch_inputs, torch_targets, torch_permanent_water, transform = read_inference_pair(tiff_s2, folder_ground_truth="/gt/",
window=window,
return_ground_truth=True, channels=channels,
folder_permanent_water="/PERMANENTWATERJRC/",
cache_folder=None)
outputs, cont_pred = inference_function(torch_inputs[0])
plot_inference_set(torch_inputs, torch_targets, outputs, torch_permanent_water, transform)
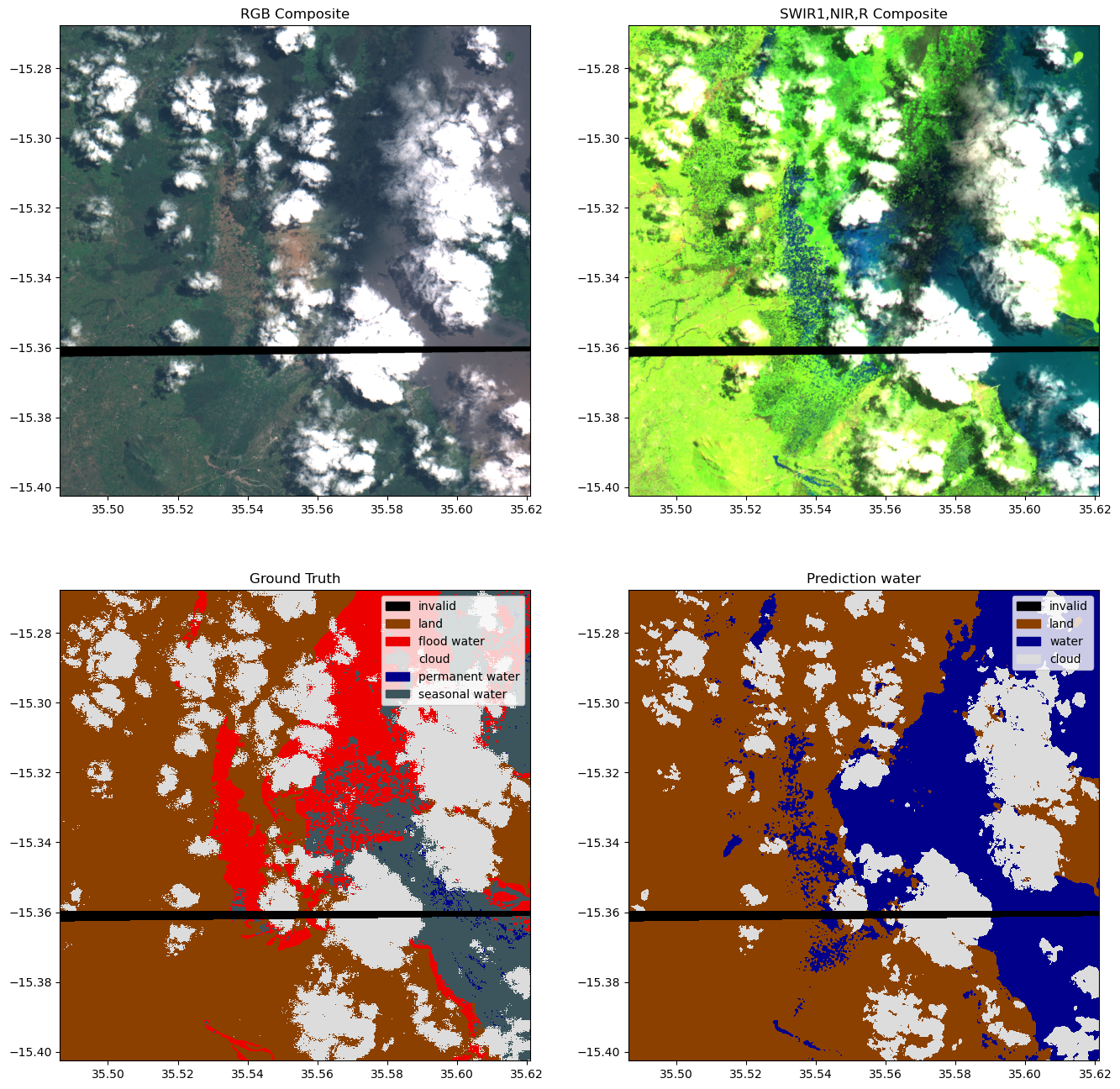
Lets try another image from the new data prepared by the Janitors!#
import rasterio.windows
window = rasterio.windows.Window(col_off=1543, row_off=247,
width=2000, height=2000)
tiff_s2, channels = "gs://ml4cc_data_lake/2_PROD/1_Staging/WorldFloods/S2/EMSR501/AOI01/EMSR501_AOI01_DEL_MONIT01_r1_v1.tif", get_channel_configuration_bands(config.model_params.hyperparameters.channel_configuration)
torch_inputs, torch_targets, torch_permanent_water, transform = read_inference_pair(tiff_s2, folder_ground_truth="/GT/V_1_1/",
window=window,
return_ground_truth=True, channels=channels,
folder_permanent_water="/JRC/",
cache_folder=cache_folder)
outputs, cont_pred = inference_function(torch_inputs[0])
plot_inference_set(torch_inputs, torch_targets, outputs, torch_permanent_water, transform)
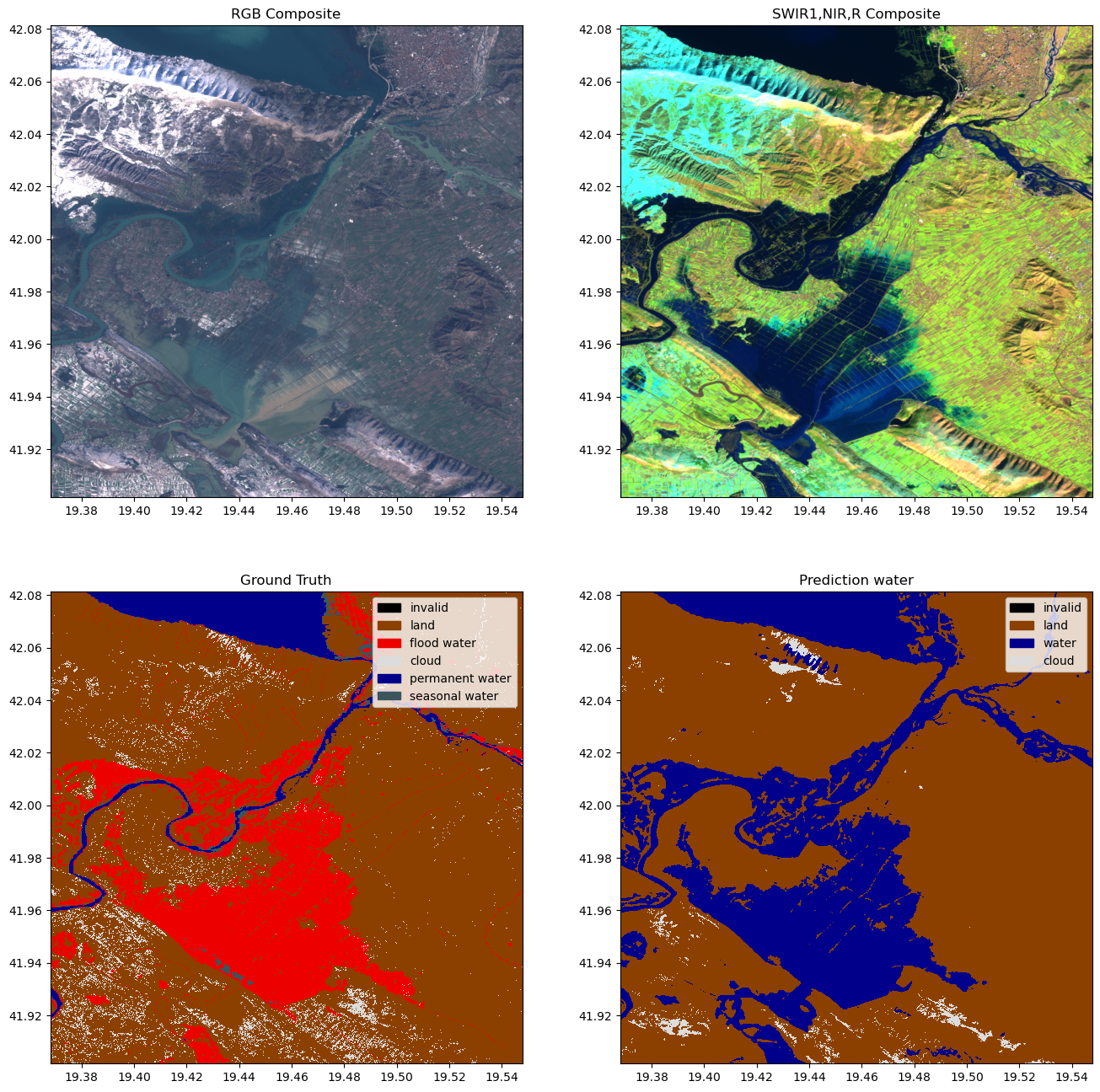
Licence#
The ML4Floods package is published under a GNU Lesser GPL v3 licence
The WorldFloods database and all pre-trained models are released under a Creative Commons non-commercial licence. For using the models in comercial pipelines written consent by the authors must be provided.
The Ml4Floods notebooks and docs are released under a Creative Commons non-commercial licence.
If you find this work useful please cite:
@article{portales-julia_global_2023,
title = {Global flood extent segmentation in optical satellite images},
volume = {13},
issn = {2045-2322},
doi = {10.1038/s41598-023-47595-7},
number = {1},
urldate = {2023-11-30},
journal = {Scientific Reports},
author = {Portalés-Julià, Enrique and Mateo-García, Gonzalo and Purcell, Cormac and Gómez-Chova, Luis},
month = nov,
year = {2023},
pages = {20316},
}
Acknowledgments#
This research has been supported by the DEEPCLOUD project (PID2019-109026RB-I00) funded by the Spanish Ministry of Science and Innovation (MCIN/AEI/10.13039/501100011033) and the European Union (NextGenerationEU).
